News
William DeGroat shines at the 19th Annual Undergraduate Research Symposium
Apr 27, 2023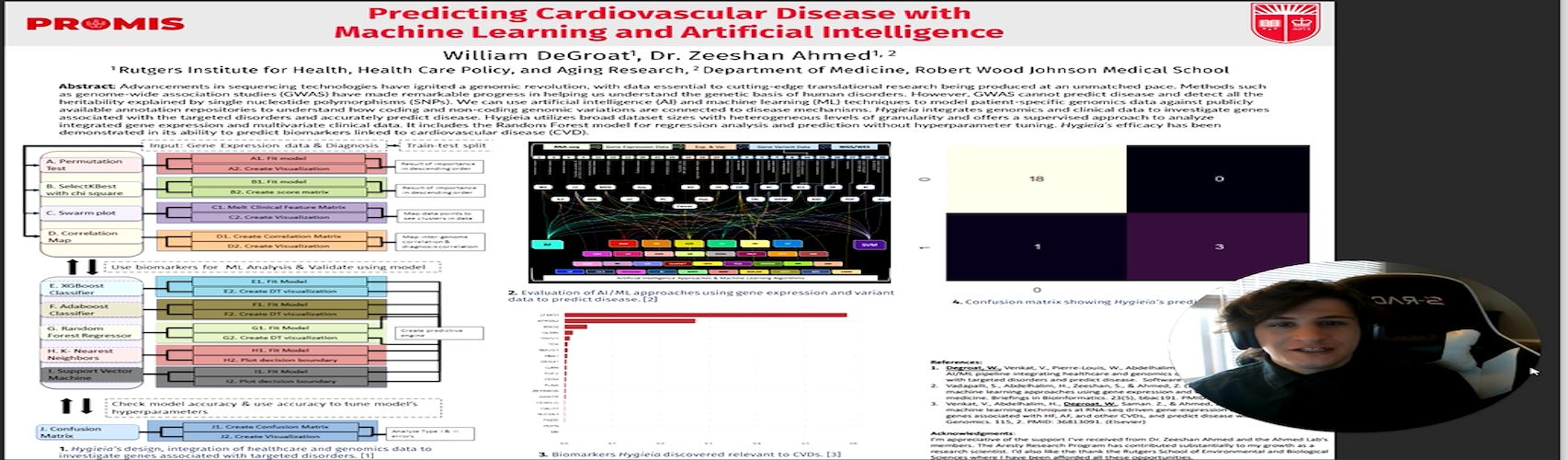
Mr. William DeGroat, presenting, “Artificial intelligence and machine learning approaches using integrated clinical and multi-omics data to predict cardiovascular disease“, at the 19th Annual Undergraduate Research Symposium-April 28, 2023, organized by the Aresty Research Center for Undergraduates, at Rutgers, The State University of New Jersey.
Summary:
Advancements in sequencing technologies have ignited a genomic revolution, with data essential to cutting-edge translational research being produced at an unmatched pace. Methods such as genome-wide association studies (GWAS) have made remarkable progress in helping us understand the genetic basis of the human disorders. However, GWAS cannot predict disease and detect all the heritability explained by single nucleotide polymorphisms (SNPs). We can use artificial intelligence (AI) and machine learning (ML) techniques to model patient-specific genomics data against publicly available annotation repositories to understand how coding and non-coding genomic variations are connected to disease mechanisms. Hygieia integrates genomics and clinical data to investigate genes associated with the targeted disorders and accurately predict disease. Hygieia utilizes broad dataset sizes with heterogeneous levels of granularity and offers a supervised approach to analyze integrated gene expression and multivariate clinical data. It includes the Random Forest model for regression analysis and prediction without hyperparameter tuning. Hygieia’s efficacy has been demonstrated in its ability to predict biomarkers linked to cardiovascular disease (CVD).
Related Publications by William:
– Degroat, W., Venkat, V., Pierre-Louis, W., Abdelhalim, H., & Ahmed, Z*. (2023). Hygieia: AI/ML pipeline integrating healthcare and genomics data to investigate genes associated with targeted disorders and predict disease. Software Impacts. 100493. (Elsevier).
– Venkat, V., Abdelhalim, H., Degroat, W., Saman. Z., & Ahmed, Z*. (2023). Implementing machine learning techniques at RNA-seq driven gene-expression data to investigate genes associated with HF, AF, and other CVDs, and predict disease with high accuracy. Genomics. 115, 2. PMID: 36813091. (Elsevier)